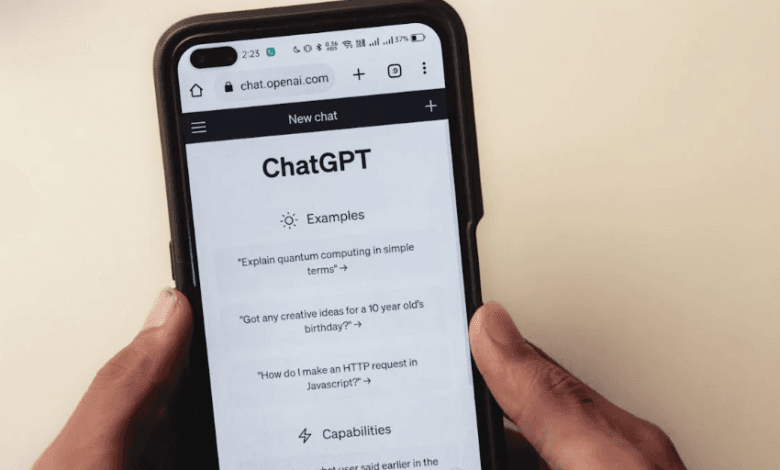
AI investors and founders referred to the “second era of scaling laws” last month, pointing out that tried-and-true techniques for enhancing AI models were yielding dwindling returns. “Test-time scaling,” a promising new technique they proposed that may maintain advances, appears to be the driving force behind OpenAI’s o3 model’s success, but it has its own set of disadvantages.
The release of OpenAI’s o3 model was interpreted by many in the AI community as evidence that the field has not “hit a wall” in its progress toward scale. The o3 model performs well on benchmarks, scoring 25% on a challenging math exam that no other AI model scored more than 2% on and vastly outperforming all other models on a general ability test known as ARC-AGI.
Noam Brown, a co-creator of OpenAI’s o-series of models, pointed out on Friday that the company is just revealing o3’s remarkable advances three months after o1 was revealed. This is a comparatively short period of time for such a performance leap.
In a tweet, Brown stated, “We have every reason to believe this trajectory will continue.”
In a blog post on Monday, Jack Clark, a co-founder of Anthropic, stated that o3 is proof that AI “will be faster in 2025 than in 2024.” (Remember that even though Clark is assisting a rival, it helps Anthropic, particularly its capacity to seek funds, to imply that AI scaling laws are still in place.)
According to Clark, in order to extract even greater benefits from AI models, the field will combine test-time scaling with conventional pre-training scaling techniques in the upcoming year. He might be implying that, like Google did last week, Anthropic and other AI model providers would reveal their own reasoning models in 2025.