Nvidia CEO Jensen Huang says his AI chips are improving faster
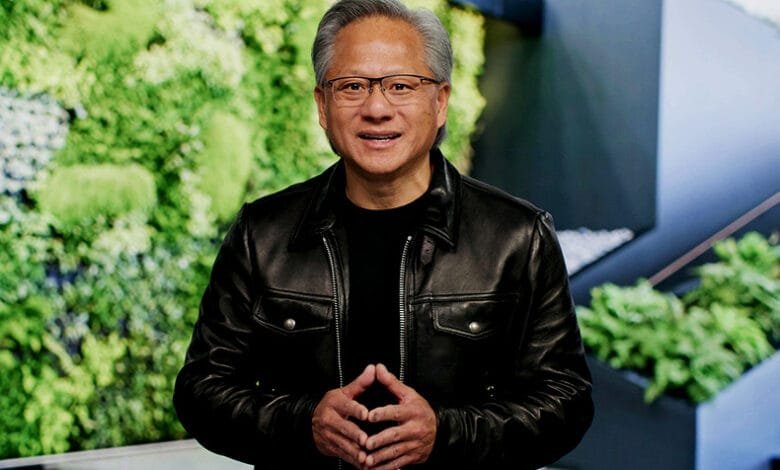
Huang said in an interview
“Our systems are progressing way faster than Moore’s Law,”
Gordon Moore, a co-founder of Intel, came up with the idea of Moore’s Law in 1965. It said that as the number of transistors on computer chips increased, the devices’ performance would essentially double annually. This forecast largely came to pass, resulting in decades of rapidly increasing capabilities and falling costs.
Moore’s Law has slowed down in recent years. Huang asserts, however, that Nvidia’s AI processors are developing at a quicker rate than their own; the company boasts that its most recent data center superchip can process AI inference workloads more than 30 times faster than its predecessor.
Huang said
“We can build the architecture, the chip, the system, the libraries, and the algorithms all at the same time,”. “If you do that, then you can move faster than Moore’s Law, because you can innovate across the entire stack.”
The CEO of Nvidia made the audacious assertion at a time when many are wondering if AI has reached a standstill. Nvidia’s AI chips are used by top AI labs like Google, OpenAI, and Anthropic to train and execute their AI models; improvements to these chips should result in even greater gains in the capabilities of AI models.
Huang has previously claimed that Nvidia is outperforming Moore’s Law. Huang stated in a November podcast that the field of artificial intelligence is headed toward “hyper Moore’s Law.”
Huang disputes the notion that advancements in AI are stalling. Rather, he asserts that there are currently three active AI scaling laws: test-time compute, which takes place during the inference phase and allows an AI model more time to “think” after each question; post-training, which refines an AI model’s responses using techniques like human feedback; and pre-training, which is the initial training phase where AI models learn patterns from vast amounts of data.
Huang also told
“Moore’s Law was so important in the history of computing because it drove down computing costs,”. “The same thing is going to happen with inference where we drive up the performance, and as a result, the cost of inference is going to be less.”